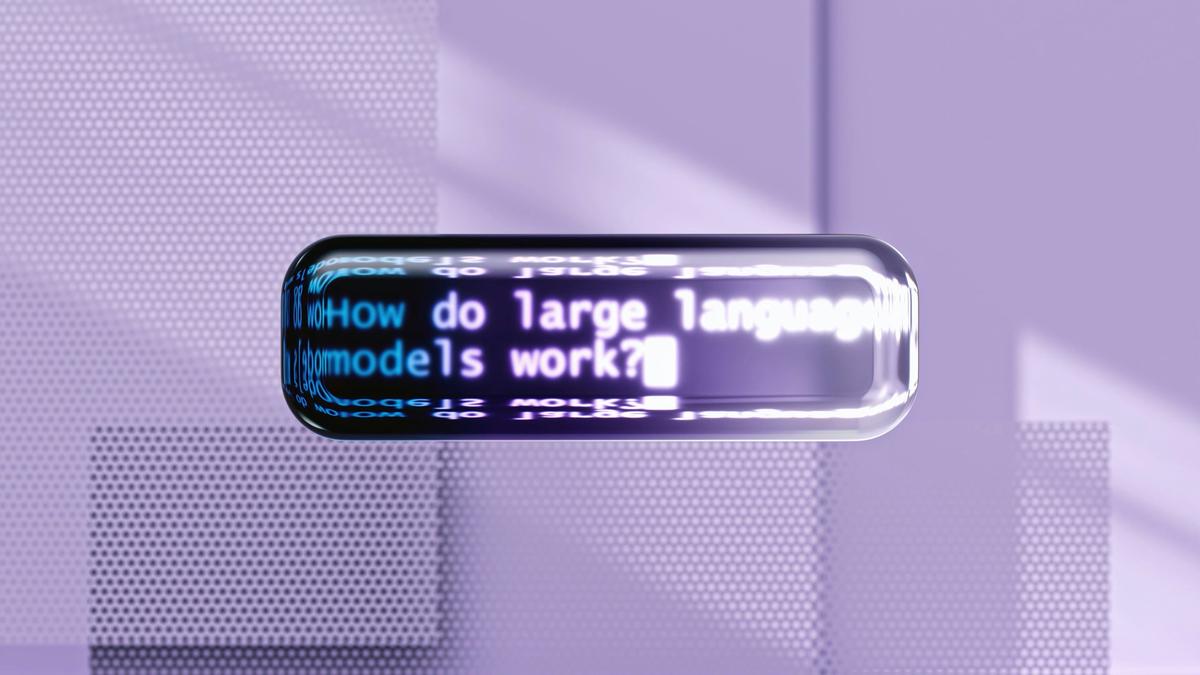
How quantum computing can make large language models even better Premium
The Hindu
Discover the transformative potential of quantum computing in revolutionizing AI applications for sustainable, efficient, and performant language models.
In recent years, the landscape of artificial intelligence (AI), particularly within the realm of natural language processing (NLP), has undergone a remarkable transformation. We have witnessed the rise of powerful large language models (LLMs) made by OpenAI, Google, and Microsoft, among others, and generative AI (Gen-AI), characterised by its unparalleled ability to generate data based on user inputs.
These sophisticated models have revolutionised human-computer interactions, bestowing upon users experiences akin to human understanding. The advent of these cutting-edge technologies and their wide availability has compelled the people at large, industry stakeholders, and governmental bodies to pay attention to their implications.
LLMs are a cornerstone in AI and mirror the complexities of human language processing. They can classify text, answer questions, and translate between languages. But they also consume a lot of energy to be trained and when put in use.
For example, as models go, LLMs are much larger than other AI applications such as computer vision. The energy consumption of a large language model (LLM) is determined mostly by the number of parameters it has. Larger models demand more computational power for both training and inference. For example, GPT-3 has 175 billion parameters and required around 1,287 MWh of electricity to train. This is around what an average American household consumes in 120 years.
LLMs also surpass non-AI applications in this regard. Training an LLM with 1.75 billion parameters can emit up to 284 tonnes of carbon dioxide, which represents more energy than that required to run a data centre with 5,000 servers for a year.
It’s important that we lower LLMs’ carbon footprint to ensure they are sustainable and cost-effective. Achieving these goals will give LLMs more room to become more sophisticated as well.
Another shortcoming of LLMs pertains to their pre-trained nature, which restricts the level of control users have over their functioning. These models are trained on large datasets with which they develop awareness of word-use patterns in diverse linguistic contexts. But such training often also results in “hallucinations”. Essentially, LLMs may generate text that is contextually coherent but factually incorrect or semantically nonsensical. This arises from limitations inherent to the training, when the model’s understanding may diverge from reality.

VinFast’s entry into India is a strategic one, and it comes with real investment. The company is setting up a manufacturing facility in Tuticorin, Tamil Nadu, with an initial capacity of 50,000 vehicles per year, scalable to 150,000 units annually. With an investment of up to USD 500 million, this is not a soft launch—it is a full-fledged push into what is one of the world’s most promising auto markets.

On June 7, 1742, Prussian mathematician Christian Goldbach wrote a letter to Swiss polymath Leonhard Euler. Why does it warrant attention, you seem to be asking. Goldbach outlined a conjecture that bears his name in this letter, a conjecture that is now one of the oldest and most famous unsolved problems in all of number theory. Intrigued? A.S.Ganesh hands you more details about Goldbach and Goldbach’s conjecture…