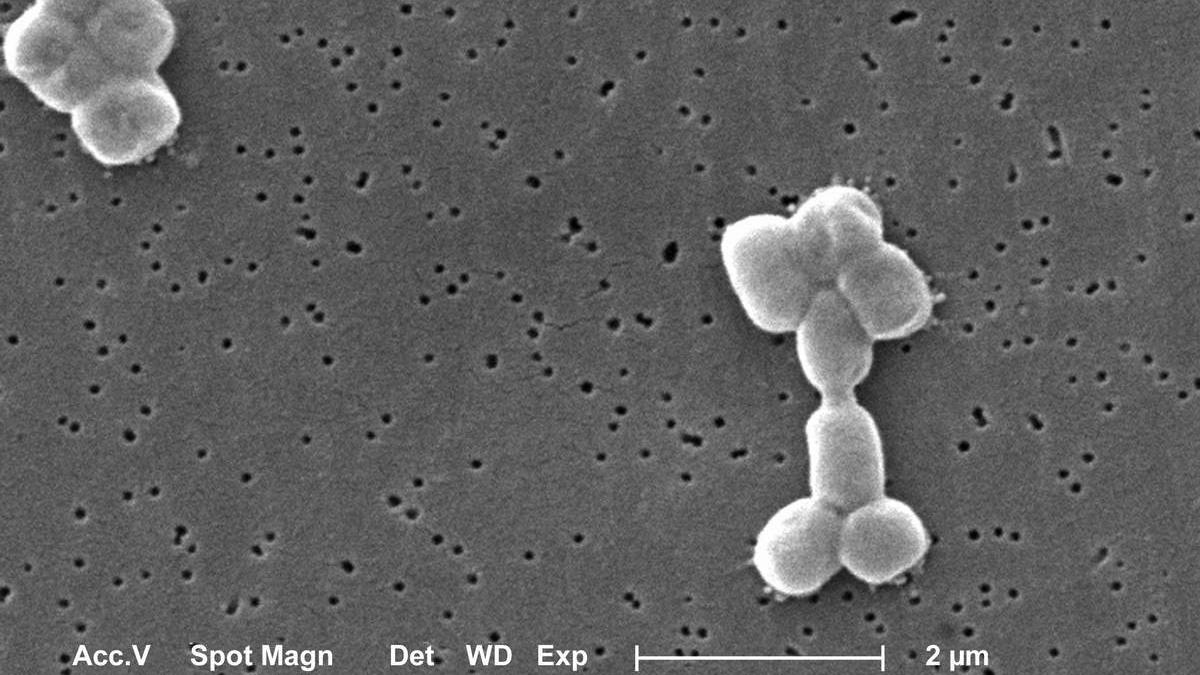
Explained | Abaucin, the potential new antibiotic found with machine-learning Premium
The Hindu
Researchers in the US and Canada have reported rediscovering a known compound called abaucin for its antibacterial properties against Acinetobacter baumannii, a notorious pathogenic bacteria known to resist multiple antibiotics, using machine learning.
Researchers have used machine-learning to identify a potential new antibiotic against a challenging species of disease-causing bacteria, they reported in a paper published in Nature Chemical Biology on May 25.
The finding is important because of the rise of antimicrobial resistance and the struggle to identify new classes of antibiotics. It also clarifies how machines can help speed up the identification, discovery, and testing of new antibiotics that the world desperately needs – and potentially reduce the cost of this laborious process.
Antimicrobial resistance is one of the great crises of the 21st century that, like climate change, was brought on by human activities and affects the whole world, regardless of borders or points of origin. It refers to the ability of microbes to evolve to resist the compounds humans have developed to beat them.
As a result, many drugs, but especially antibiotics, have become less effective or ineffective against disease-causing bacteria, allowing the diseases to become more prevalent again.
The global cost of antimicrobial resistance is expected to be $300 billion to more than $1 trillion every year. India is a ‘hotspot’ of antimicrobial resistance thanks to the overuse of antibiotics, among people and animals, and the improper disposal of pharmaceutical waste.
Efforts to develop new antibiotics have been hamstrung by the fact that many existing compounds have been derived from a smaller group. This implies a higher cost and longer timelines to identify new drugs that can push back the tide of resistance.
One promising pathway here is to use machine-learning models that can be ‘taught’ to look for molecules with properties considered desirable to fight specific species of bacteria. Such models can also sift through large datasets in a short duration.